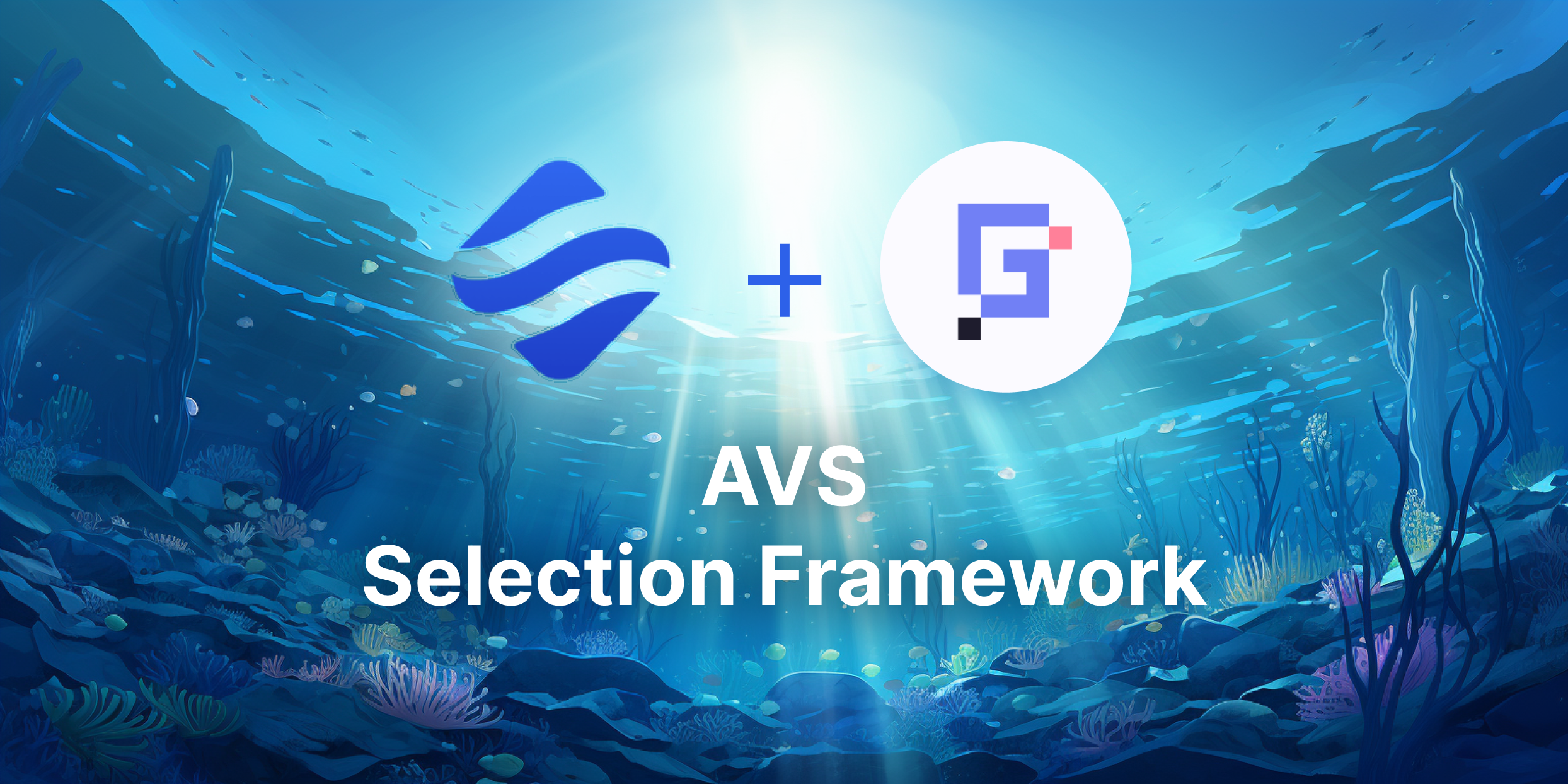
Abstract
Liquid restaking is quickly becoming one of the most important developments in decentralized finance. The rapid rise of liquid restaking token (LRT) adoption and growth was made possible by EigenLayer, a protocol built on Ethereum, that extends Ethereum security through restaking. Liquid restaking involves the liquification of restaked liquidity deposited on EigenLayer which will soon be used to provide pooled security to Actively Validated Services (AVSs) which are services, such as bridges, oracles, L2s, and more which require decentralized trust.
Swell DAO, a liquid restaking protocol, is developing an LRT, rswETH. As part of the DAO’s commitment to risk management, Swell engaged Gauntlet early in the continuous development of rswETH. In this paper, Gauntlet proposes a strategy framework for the selection of AVS's with consideration to factors such as the fee generation, penalty, and the overall dynamic and evolving state of EigenLayer and the overall AVS ecosystem.
Introduction
As part of a recent collaboration between Swell DAO and Gauntlet, Swell tasked Gauntlet with devising a mathematical framework for selecting AVS's to be included in their rswETH Liquid Restaked Token (LRT). Although much information still remains unknown regarding the AVS ecosystem and EigenLayer’s implementation, the high-level framework led by Gauntlet provides a starting point for LRT design. For those interested in a deeper mathematical framework, see the full report, but the below blog summarizes the framework and how it can be incorporated into rswETH.
Proposed design for rswETH v2
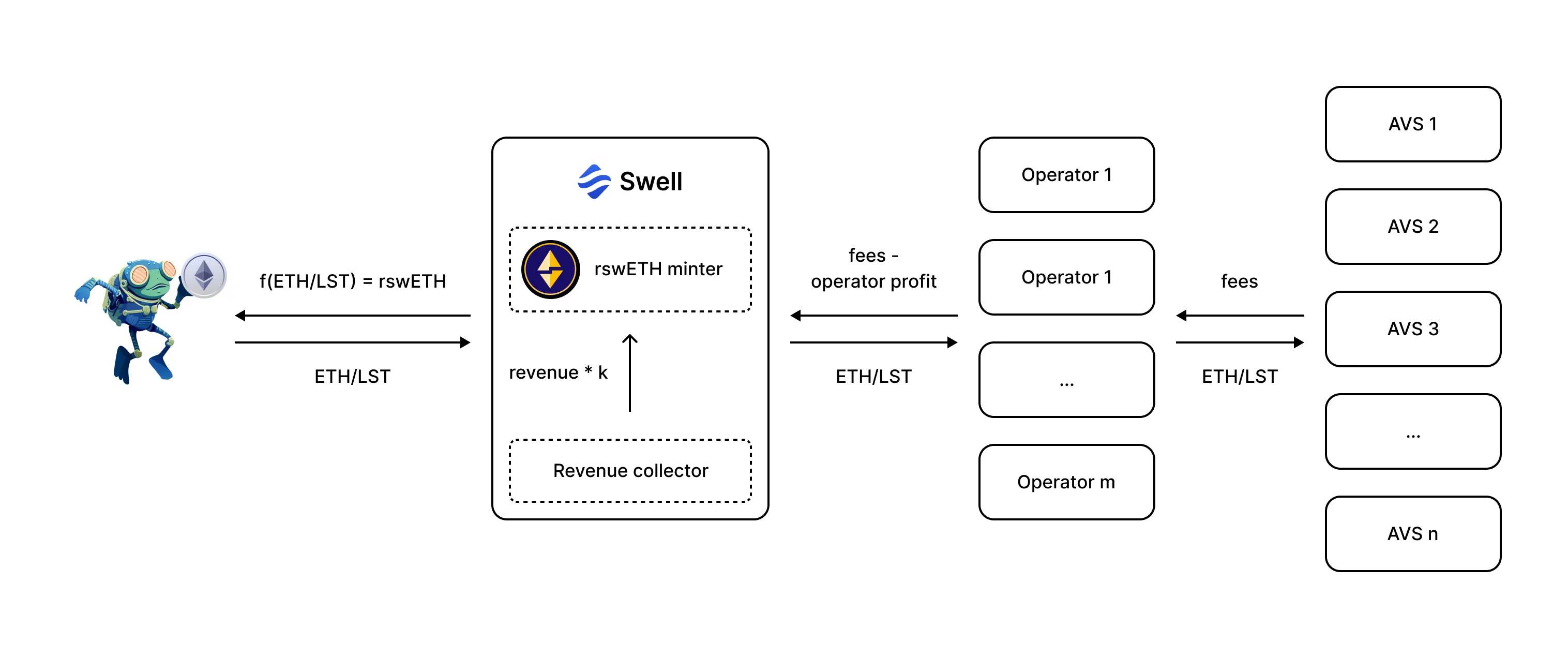
On AVS Selection
A general approach for evaluating a group of AVSs against each other is to seek maximum risk-adjusted total returns. In our approach, this is quantified as fee generation less the expected slashing over some future time period. Further, given that AVS yield will likely be variable, time periods are broken up into rebalancing periods to adjust throughout the LRT's lifecycle.
For simplicity, we used a 2 week rebalance period since it’s double the AVS withdrawal period. When an LRT decides to implement the framework, this rebalance period can be further optimized based on AVS behavior. By designating a rebalance period, it allows us to compare projected yields from current AVS's to future AVS's post-rebalancing.
The AVS selection model must also consider the LRT's size. This is likely self-explanatory, but the allocation to a single AVS cannot be greater than the LRT's TVL (duh!). This constraint, and other similar constraints, are all incorporated into the mathematical model.
The model can then be converted into an “objective function”, which when solved, will output the optimal risk-adjusted allocation of AVS's. This objective function will allow Gauntlet to provide specific allocation recommendations while incorporating an LRT’s risk preferences!
AVS Level Considerations
Beyond AVS selection, rswETH can incorporate additional considerations at the AVS level to adjust beyond the optimization. The bounds described below aim to contain the downsides associated with a particular AVS and enforce a baseline profitability.
- Each AVS has a bounded slashing probability: The maximum amount that can be slashed by a single AVS should be less than the minimum expected payout from that AVS.
- AVS payout is positive in isolation: disregarding potential correlation with other AVSs, The expected payout of a single AVS should be positive.
- Correlated slashing is bounded: The correlated slashing potential is minimized below some appetite defined by Swell.
Iterative Performance
The final piece of the mathematical framework defines considerations for iterative performance throughout multiple rebalancing cycles.
Performance in previous rebalance windows may give information on how future optimization could be improved, such as adjusting constraints or adding additional filtering. This can be accomplished by ensuring a rolling Sharpe ratio remains above a minimum Sharpe benchmark defined by the Swell DAO in accordance with governance mechanisms.
Conclusion
We lay out a mathematical framework above on how to approach AVS selection. Fundamentally, allocations among different AVS's is rooted in our estimation for net yields and slashing from those AVS's, with global constraints from Eigenlayer mechanisms. Based on risk aversion, we may also impose a number of conditions that filter AVS's on a more granular level.
The lack of formalized information regarding AVS slashing conditions can make it difficult to generate specific recommendations on stake allocation among different AVSs. Nonetheless, we seek to find generalized principles around AVS allocation that can remain robust.
The question that naturally follows is: How do we capture the inherently developing nature of restaking? This dynamic relationship between restakers, the marketplace, and the AVSs — and how they interact with natural market variance and shocks — suggests that careful agent-based simulation can find that intricate balance between restaking yield and AVS slashing penalties, and ultimately drive greater capital efficiency.
Stay up-to-date with Swell by joining us in Discord and following us on Farcaster, Twitter and DeBank.